Mastering Statistical Significance and Sample Size Calculation for A/B Tests
Understanding the crucial aspects of statistical significance and sample size calculation is essential for successful A/B testing in conversion rate optimization.
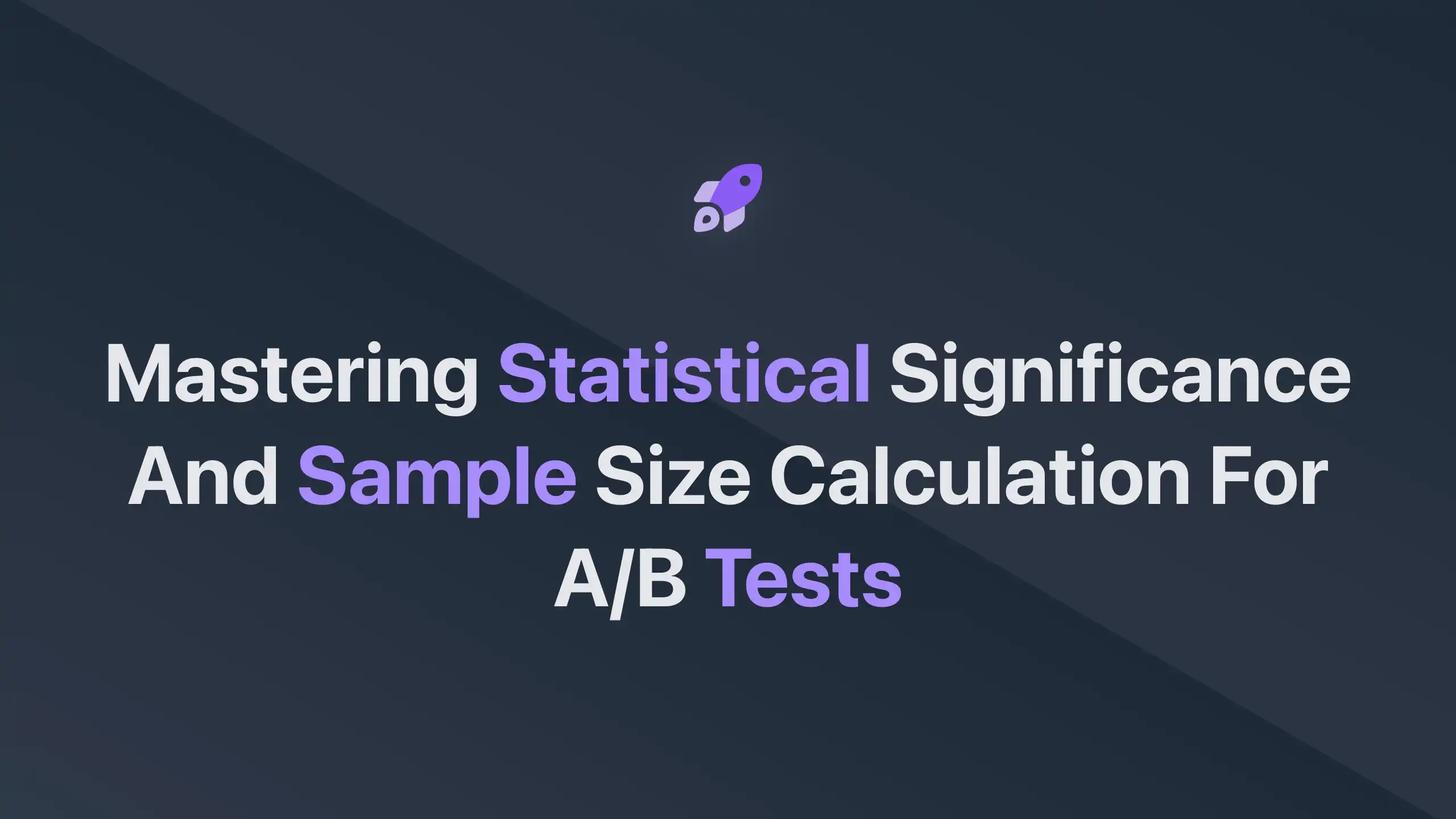
The Importance of Statistical Significance in A/B Testing
In the world of conversion rate optimization, A/B testing is a powerful tool used to determine which version of a webpage or app leads to better conversion rates. However, the results of A/B tests are only meaningful if they are statistically significant. Statistical significance ensures that the observed differences between the variants are not due to random chance.
What is Statistical Significance?
Statistical significance is a measure of the likelihood that the differences observed in the A/B test are real and not just a result of random variation. It helps us determine whether the changes made to a webpage or app actually have an impact on conversion rates.
How to Determine Statistical Significance?
To determine statistical significance, we can use hypothesis testing and calculate the p-value. The p-value indicates the probability of obtaining the observed results by random chance alone. A p-value below a certain threshold (usually 0.05) indicates that the results are statistically significant.
Sample Size Calculation for A/B Tests
One of the key factors that influence the statistical significance of an A/B test is the sample size. A larger sample size reduces the margin of error and increases the likelihood of detecting a true difference between the variants.
Why is Sample Size Important?
A small sample size can lead to unreliable results and false conclusions. It is crucial to calculate the sample size needed for an A/B test before conducting the experiment to ensure the results are statistically meaningful.
How to Calculate Sample Size?
There are various methods for calculating sample size for A/B tests, including the formula based on statistical power, effect size, and significance level. Tools like online calculators can also help determine the appropriate sample size for an A/B test.
Sample size calculation is a critical step in ensuring the validity and reliability of A/B test results.
Understanding statistical significance and sample size calculation is essential for conducting successful A/B tests in conversion rate optimization. By ensuring the results are statistically meaningful, businesses can make informed decisions to improve their conversion rates and drive growth.
Unlock the full potential of your landing page with ConvertRocket.ai. This affordable AI-powered conversion rate optimization platform can help you skyrocket your landing page's conversion rates with actionable insights and personalized examples. Try ConvertRocket.ai today!